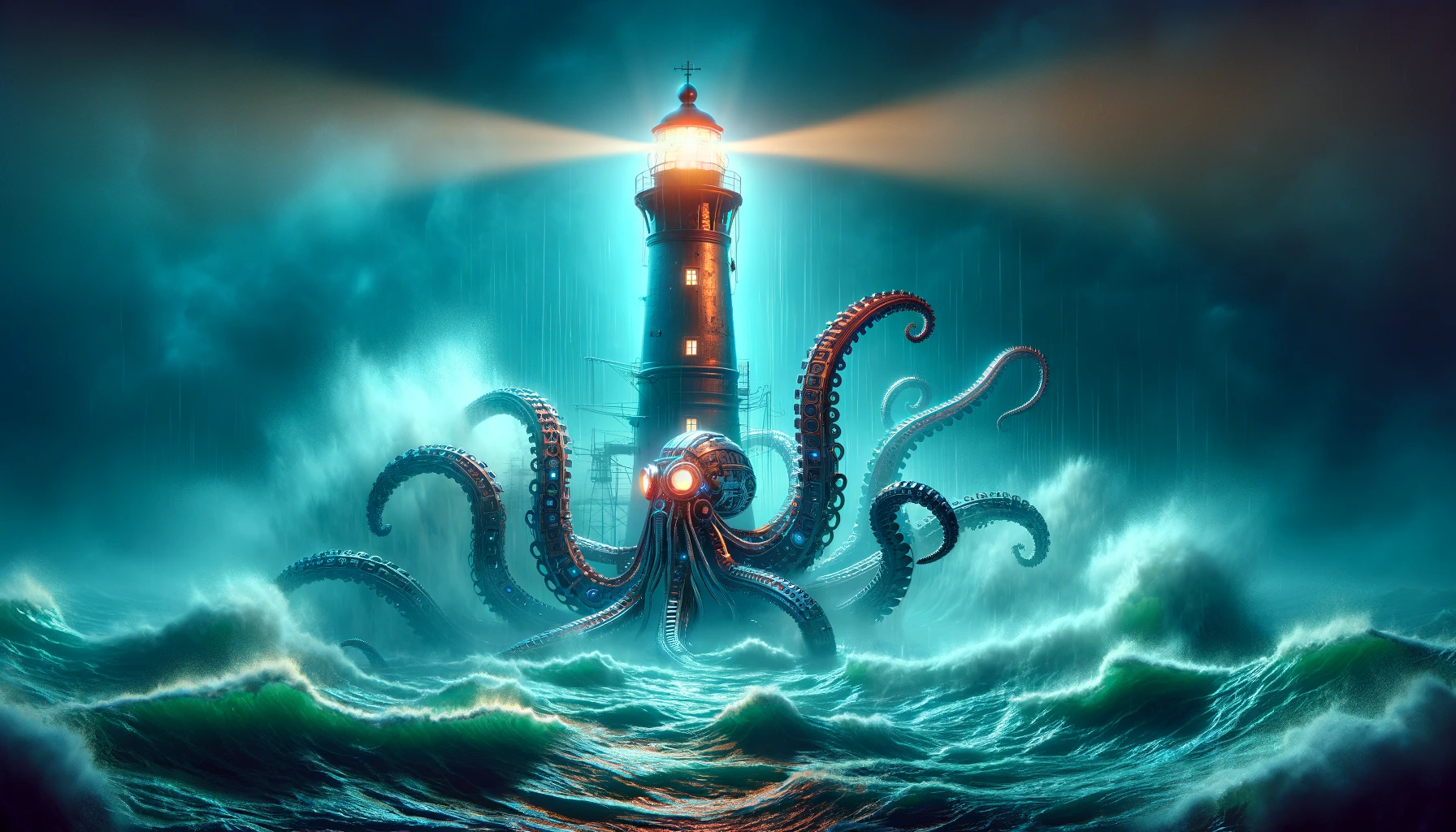
This series will investigate the phenomenon of Attentional Energy, and why it drives intelligent agents, natural born or otherwise created. The Framework of Attention that I use is Memetics. It will be crucial to understand why biological evolution switched from vertical, hereditary evolution and mutation mechanisms to horizontal, memetic means of information transportation and why the brain and its neural content became the motor of this evolution. In later Episodes I will show why Simulations are crucial and why it is no mere coincidence that the most productive playground for technological and other innovation is founded in the excessive Game Drive of higher mammals.

Short Introduction to Memes and Tokens
Survival machines that can simulate the future are one jump ahead of survival machines that who can only learn of the basis of trial and error. The trouble with overt trial is that it takes time and energy. The trouble with overt error is that it is often fatal…. The evolution of the capacity to simulate seems to have culminated in subjective consciousness. Why this should have happened is, to me, the most profound mystery facing modern biology.
Ch. 4. The Gene machine – The Selfish Gene (1976, 1989)
“The Selfish Gene,” authored by Richard Dawkins and first published in 1976, is a seminal work that popularized the gene-centered view of evolution. Dawkins argues that the fundamental unit of selection in evolution is not the individual organism, nor the group or species, but the gene. He proposes that genes, as the hereditary units, are “selfish” in that they promote behaviors and strategies that maximize their own chances of being replicated. Through this lens, organisms are viewed as vehicles or “survival machines” created by genes to ensure their own replication and transmission to future generations.
Dawkins introduces the concept of the “meme” as a cultural parallel to the biological gene. Memetics, as defined by Dawkins, is the theoretical framework for understanding how ideas, behaviors, and cultural phenomena replicate and evolve through human societies. Memes are units of cultural information that propagate from mind to mind, undergoing variations, competition, and inheritance much like genes do within biological evolution. This concept provides a mechanism for understanding cultural evolution and how certain ideas or behaviors spread and persist within human populations.
Dawkins’s exploration of memetics suggests that just as the survival and reproduction of genes shape biological evolution, memes influence the evolution of cultures by determining which ideas or practices become widespread and which do not. The implications of this theory extend into various fields, including anthropology, sociology, and psychology, offering insights into human behavior, cultural transmission, and the development of societies over time.

Tokens in the context of language models, such as those used in GPT-series models, represent the smallest unit of processing. Text input is broken down into tokens, which can be words, parts of words, or even punctuation, depending on the tokenization process. These tokens are then used by the model to understand and generate text. The process involves encoding these tokens into numerical representations that can be processed by neural networks. Tokens are crucial for the operation of language models as they serve as the basic building blocks for understanding and generating language.
Memes encompass ideas, behaviors, styles, or practices that spread within a culture. The meme concept is analogous to the gene in that memes replicate, mutate, and respond to selective pressures in the cultural environment, thus undergoing a type of evolution by natural selection. Memes can be anything from melodies, catch-phrases, fashion, and technology adoption, to complex cultural practices. Dawkins’ main argument was that just as genes propagate by leaping from body to body via sperm or eggs, memes propagate by leaping from brain to brain.
Tokens in the context of language models, such as those used in GPT-series models, represent the smallest unit of processing. Text input is broken down into tokens, which can be words, parts of words, or even punctuation, depending on the tokenization process. These tokens are then used by the model to understand and generate text. The process involves encoding these tokens into numerical representations that can be processed by neural networks. Tokens are crucial for the operation of language models as they serve as the basic building blocks for understanding and generating language.
Both memes and tokens act as units of transmission in their respective domains. Memes are units of cultural information, while tokens are units of linguistic information.
There are also differences.

Memes evolve through cultural processes as they are passed from one individual to another, adapting over time to fit their cultural environment. Tokens, however, do not evolve within the model itself; they are static representations of language used by the model to process and generate text. The evolution in tokens can be seen in the development of better tokenization techniques and models over time, influenced by advancements in the field rather than an adaptive process within a single model.
Memes replicate by being copied from one mind to another, often with variations. Tokens are replicated exactly in the processing of text but can vary in their representation across different models or tokenization schemes.
The selection process for memes involves cultural acceptance , relevance, and transmission efficacy, leading to some memes becoming widespread while others fade. For tokens, the selection process is more about their effectiveness in improving model performance, leading to the adoption of certain tokenization methods over others based on their ability to enhance understanding or generation of language. In the selection process during training tokens are weighed by other human minds (meme machines) and selected for attraction, token pools that are better liked have a higher probabilistic chance of occurring.
Memeplexes can be complex and abstract, encompassing a wide range of cultural phenomena, but all the memes which they contain are very simple and elementary.
Tokens are generally even simpler, representing discrete elements of language, though the way these tokens are combined and used by the model can represent complex ideas.

The title of the Google paper Attention is All You Need is a bold statement that reflects a significant shift in the approach to designing neural network architectures for natural language processing (NLP) and beyond. Published in 2017 by Vaswani et al., this paper introduced the Transformer model, which relies heavily on the attention mechanism to process data. The term “attention” in this context refers to a technique that allows the model to focus on different parts of the input data at different times, dynamically prioritizing which aspects are most relevant for the task at hand.
Before the advent of the Transformer model, most state-of-the-art NLP models were based on recurrent neural networks (RNNs) or convolutional neural networks (CNNs), which processed data sequentially or through local receptive fields, respectively. These approaches had limitations, particularly in handling long-range dependencies within the data (e.g., understanding the relationship between two words far apart in a sentence).
The attention mechanism, as utilized in the Transformer, addresses these limitations by enabling the model to weigh the significance of different parts of the input data irrespective of their positions. This is achieved through self-attention layers that compute representations of the input by considering how each word relates to every other word in the sentence, allowing the model to capture complex dependencies and relationships within the data efficiently.
The key innovation of the Transformer and the reason behind the paper’s title is the exclusive use of attention mechanisms, without reliance on RNNs or CNNs, to process data. This approach proved to be highly effective, leading to significant improvements in a wide range of NLP tasks, such as machine translation, text summarization, and many others. It has since become the foundation for subsequent models and advancements in the field, illustrating the power and versatility of attention mechanisms in deep learning architectures.
There is a point to be made that this kind of attention is the artificial counterpart to the natural instinct of love that binds mammal societies. Which would mean that the Beatles were right after all.
An in-formation that causes a trans-formation
What we mean by information — the elementary unit of information — is a difference which makes a difference, and it is able to make a difference because the neural pathways along which it travels and is continually transformed are themselves provided with energy. The pathways are ready to be triggered. We may even say that the question is already implicit in them.
p. 459, Chapter “Form, Substance and Difference” – Steps to an Ecology of Mind (1972)
The Transformer architecture was already hinted at by Bateson in 1972, decades before we knew about neural plasticity.
Bateson’s idea revolves around the concept that information is fundamentally a pattern or a difference that has an impact on a system’s state or behavior. For Bateson, not all differences are informational; only those that lead to some form of change or response in a given context are considered as conveying information. This perspective is deeply rooted in cybernetics and the study of communication processes in and among living organisms and machines.
The quote “a difference that makes a difference” encapsulates the notion that information should not be viewed merely as data or raw inputs but should be understood in terms of its capacity to influence or alter the dynamics of a system. It’s a foundational concept in understanding how information is processed and utilized in various systems, from biological to artificial intelligence networks, emphasizing the relational and contextual nature of information.
This concept has far-reaching implications across various fields, including psychology, ecology, systems theory, and artificial intelligence. It emphasizes the relational and contextual nature of information, suggesting that the significance of any piece of information can only be understood in relation to the system it is a part of. For AI and cognitive science, this principle underscores the importance of context and the interconnectedness of information pathways in understanding and designing intelligent systems.
Hinton, Sutskever and others consistently argue that for models like GPT 4.0 to achieve advanced levels of natural language processing (NLP), they must truly grasp the content with which they are dealing. This understanding comes from analyzing vast amounts of digital data created by humans, allowing these models to form a realistic view of the world from a human perspective. Far from being mere “stochastic parrots” as sometimes depicted by the media, these models offer a more nuanced and informed reflection of human knowledge and thought processes.