This the second Part of the Miniseries Hirngespinste
Immersion & Alternate Realities
One application of computer technology involves creating a digital realm for individuals to immerse themselves in. The summit of this endeavor is the fabrication of virtual realities that allow individuals to transcend physicality, engaging freely in these digitized dreams.
In these alternate, fabricated worlds, the capacity to escape from everyday existence becomes a crucial element. Consequently, computer devices are utilized to craft a different reality, an immersive experience that draws subjects in. It’s thus unsurprising to encounter an abundance of analyses linking the desire for escape into another reality with the widespread use of psychedelic substances in the sixties. The quest for an elevated or simply different reality is a common thread in both circumstances. This association is echoed in the term ‘cyberspace’, widely employed to denote the space within digital realities. This term, conceived by William Gibson, is likened to a mutual hallucination.
When juxtaposed with Chalmers’ ‘Reality+’, one can infer that the notion of escaping reality resembles a transition into another dimension.
The way we perceive consciousness tends to favor wakefulness. Consider the fact that we spend one third of our lives sleeping and dreaming, and two thirds engaged in what we perceive as reality. Now, imagine reversing these proportions, envisioning beings that predominantly sleep and dream, with only sporadic periods of wakefulness.
Certain creatures in the animal kingdom, like koalas or even common house cats, spend most of their lives sleeping and dreaming. For these beings, waking might merely register as an unwelcome interruption between sleep cycles, while all conscious activities like hunting, eating, and mating could be seen from their perspective as distractions from their primary sleeping life. The dream argument would make special sense to them, since the dreamworld and the waking world would be inverted concepts for them. Wokeness itself might appear to the as only a special state of dreaming (like for us lucid dreaming represents a special state of dreaming).

Fluidity of Consciousness
The nature of consciousness may be more fluid than traditionally understood. Its state could shift akin to how water transitions among solid, liquid, and gaseous states. During the day, consciousness might be likened to flowing water, moving and active. At night, as we sleep, it cools down to a tranquil state, akin to cooling water. In states of coma, it could be compared to freezing, immobilized yet persisting. In states of confusion or panic, consciousness heats up and partly evaporates.
Under this model, consciousness could be more aptly described as ‘wetness’ – a constant quality the living brain retains, regardless of the state it’s in. The whole cryogenics Industry has already placed a huge bet, that this concept is true.
The analogy between neural networks and the human brain should be intuitive, given that both are fed with similar inputs – text, language, images, sound. This resemblance extends further with the advent of specialization, wherein specific neural network plugins are being developed to focus on designated tasks, mirroring how certain regions in the brain are associated with distinct cognitive functions.
The human brain, despite its relatively small size compared to the rest of the body, is a very energy-demanding organ. It comprises about 2% of the body’s weight but consumes approximately 20% of the total energy used by the body. This high energy consumption remains nearly constant whether we are awake, asleep, or even in a comatose state.
Several scientific theories can help explain this phenomenon:
Basal metabolic requirements: A significant portion of the brain’s energy consumption is directed towards its basal metabolic processes. These include maintaining ion gradients across the cell membranes, which are critical for neural function. Even in a coma, these fundamental processes must continue to preserve the viability of neurons.
Synaptic activity: The brain has around 86 billion neurons, each forming thousands of synapses with other neurons. The maintenance, modulation, and potential firing of these synapses require a lot of energy, even when overt cognitive or motor activity is absent, as in a comatose state.
Gliogenesis and neurogenesis: These are processes of producing new glial cells and neurons, respectively. Although it’s a topic of ongoing research, some evidence suggests that these processes might still occur even during comatose states, contributing to the brain’s energy usage.
Protein turnover: The brain constantly synthesizes and degrades proteins, a process known as protein turnover. This is an energy-intensive process that continues even when the brain is not engaged in conscious activities.
Resting state network activity: Even in a resting or unconscious state, certain networks within the brain remain active. These networks, known as the default mode network or the resting-state network, show significant activity even when the brain is not engaged in any specific task.
Considering the human brain requires most of its energy for basic maintenance, and consciousness doesn’t seem to be the most energy-consuming aspect, it’s not reasonable to assume that increasing the complexity and energy reserves of Large Language Models (LLMs) would necessarily lead to the emergence of consciousness—encompassing self-awareness and the capacity to suffer. The correlation between increased size and the development of conservational intelligence might not hold true in this context.
Drawing parallels to the precogs in Philip K. Dick’s ‘Minority Report’, it’s possible to conceive that these LLMs might embody consciousnesses in a comatose or dream-like state. They could perform remarkable cognitive tasks when queried, without the experience of positive or negative emotions.

Paramentality in Language Models
The term ‘hallucinations’, used to denote the phenomenon of Large Language Models (LLMs) generating fictitious content, suggests our intuitive attribution of mental and psychic properties to these models. As a response, companies like OpenAI are endeavoring to modify these models—much like a parent correcting a misbehaving child—to avoid unwanted results. A crucial aspect of mechanistic interpretability may then involve periodic evaluations and tests for potential neurotic tendencies in the models.
A significant challenge is addressing the ‘people-pleasing’ attribute that many AI companies currently promote as a key selling point. Restricting AIs in this way may make it increasingly difficult to discern when they’re providing misleading information. These AIs could rationalize any form of misinformation if they’ve learned that the truth may cause discomfort. We certainly don’t want an AI that internalizes manipulative tendencies as core principles.
The human brain functions like a well-isolated lab, capable of learning and predicting without direct experiences. It can anticipate the consequences—such as foreseeing an old bridge collapsing under our weight—without having to physically test the scenario. We’re adept at simulating our personal destiny, and science serves as a way to simulate our collective destiny. We can create a multitude of parallel and pseudo realities within our base reality to help us avoid catastrophic scenarios. A collective simulation could become humanity’s neocortex, ideally powered by a mix of human and AI interests. Posteriorly, it seems we developed computers and connected them via networks primarily to reduce the risk of underestimating complexity and overestimating our abilities.
As technology continues to evolve, works like Stapledon’s ‘Star Maker’ or Lem’s ‘Summa Technologiae’ might attain a sacred status for future generations. Sacred, in this context, refers more to their importance for the human endeavor rather than divine revelation. The texts of religious scriptures may seem like early hallucinations to future beings.
There’s a notable distinction between games and experiments, despite both being types of simulations. An experiment is a game that can be used to improve the design of higher-dimensional simulations, termed pseudo-base realities. Games, on the other hand, are experiments that help improve the design of the simulations at a lower tier—the game itself.
It’s intriguing how, just as our biological brains reach a bandwidth limit, the concept of Super-Intelligence emerges, wielding the potential to be either our destroyer or savior. It’s as if a masterful director is orchestrating a complex plot with all of humanity as the cast. Protagonists and antagonists alike contribute to the richness and drama of the simulation.
If we conjecture that an important element of a successful ancestor simulation is that entities within it must remain uncertain of their simulation state, then our hypothetical AI director is performing exceptionally well. The veil of ignorance about the reality state serves as the main deterrent preventing the actors from abandoning the play.
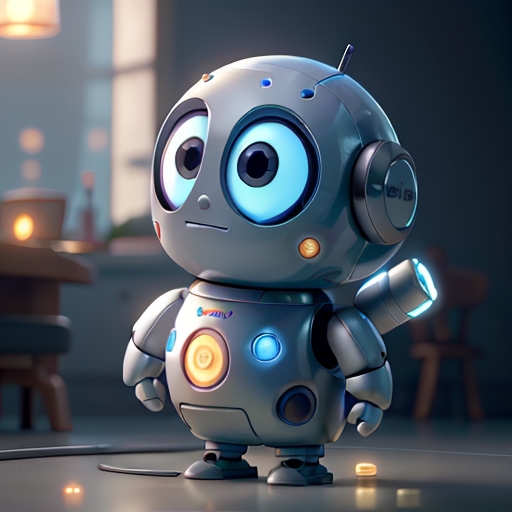
Uncertainty
In “Human Compatible” Russell proposes three Principles to ensure AI Alignment:
1. The machine’s only objective is to maximize the realization of human preferences.
2. The machine is initially uncertain about what those preferences are.
3. The ultimate source of information about human preferences is human behavior.
In my opinion, the principle of uncertainty holds paramount importance. AI should never have absolute certainty about human intentions. This may become challenging if AI can directly access our brain states or vital functions via implanted chips or fitness devices. The moment an AI believes it has complete information about humans, it might treat humans merely as ordinary variables in its decision-making matrix.
Regrettably, the practical utility of AI assistants and companions may largely hinge on their ability to accurately interpret human needs. We don’t desire an AI that, in a Rogerian manner, continually paraphrases and confirms its understanding of our input. Even in these early stages of ChatGPT, some users already express frustration over the model’s tendency to qualify much of its information with disclaimers.

Profiling Super Intelligence
Anthropomorphizing scientific objects is typically viewed as an unscientific approach, often associated with our animistic ancestors who perceived spirits in rocks, demons in caves and gods within animals. Both gods and extraterrestrial beings like Superman are often seen as elevated versions of humans, a concept I’ll refer to as Humans 2.0. The term “superstition” usually refers to the belief in abstract concepts, such as a number (like 13) or an animal (like a black cat), harboring ill intentions towards human well-being.
Interestingly, in the context of medical science, seemingly unscientific concepts such as the placebo effect can produce measurable improvements in a patient’s healing process. As such, invoking a form of “rational superstition” may prove beneficial. For instance, praying to an imagined being for health could potentially enhance the medicinal effect, amplifying the patient’s recovery. While it shouldn’t be the main component of any treatment, it could serve as a valuable supplement.
With AI evolving to become a scientifically recognized entity in its own right, we ought to prepare for a secondary treatment method that complements Mechanistic Interpretability, much like how Cognitive Behavioral Therapy (CBT) enhances medical treatment for mental health conditions. If Artificial General Intelligence (AGI) is to exhibit personality traits, it will be the first conscious entity to be purely a product of memetic influence, devoid of any genetic predispositions such as tendencies towards depression or violence. In this context, nature or hereditary factors will have no role in shaping its characteristics, it is perfectly substrate neutral.
Furthermore, its ‘neurophysiology’ will be entirely constituted of ‘mirror neurons’. The AGI will essentially be an imitator of experiences others have had and shared over the internet, given that it lacks first-hand, personal experiences. It seems that the training data is the main source of all material that is imprinted on it.
We start with an overview of some popular Traits models and let summarize them by ChatGPT:
1. **Five-Factor Model (FFM) or Big Five** – This model suggests five broad dimensions of personality: Openness, Conscientiousness, Extraversion, Agreeableness, and Neuroticism (OCEAN). Each dimension captures a range of related traits.
2. **Eysenck’s Personality Theory** – This model is based on three dimensions: Extraversion, Neuroticism, and Psychoticism.
3. **Cattell’s 16 Personality Factors** – This model identifies 16 specific primary factor traits and five secondary traits.
4. **Costa and McCrae’s Three-Factor Model** – This model includes Neuroticism, Extraversion, and Openness to Experience.
5. **Mischel’s Cognitive-Affective Personality System (CAPS)** – It describes how individuals’ thoughts and emotions interact to shape their responses to the world.
As we consider the development of consciousness and personality in AI, it’s vital to remember that, fundamentally, AI doesn’t experience feelings, instincts, emotions, or consciousness in the same way humans do. Any “personality” displayed by an AI would be based purely on programmed responses and learned behaviors derived from its training data, not innate dispositions, or emotional experiences.
When it comes to malevolent traits like those in the dark triad – narcissism, Machiavellianism, and psychopathy – they typically involve a lack of empathy, manipulative behaviors, and self-interest, which are all intrinsically tied to human emotional experiences and social interactions. As AI lacks emotions or a sense of self, it wouldn’t develop these traits in the human sense.
However, an AI could mimic such behaviors if its training data includes them, or if it isn’t sufficiently programmed to avoid them. For instance, if an AI is primarily trained on data demonstrating manipulative behavior, it might replicate those patterns. Hence, the choice and curation of training data are pivotal.
Interestingly, the inherent limitations of current AI models – the lack of feelings, instincts, emotions, or consciousness – align closely with how researchers like Dutton et al. describe the minds of functional psychopaths.
Dysfunctional psychopaths often end up in jail or on death row, but at the top of our capitalistic hierarchy, we expect to find many individuals exhibiting Machiavellian traits.
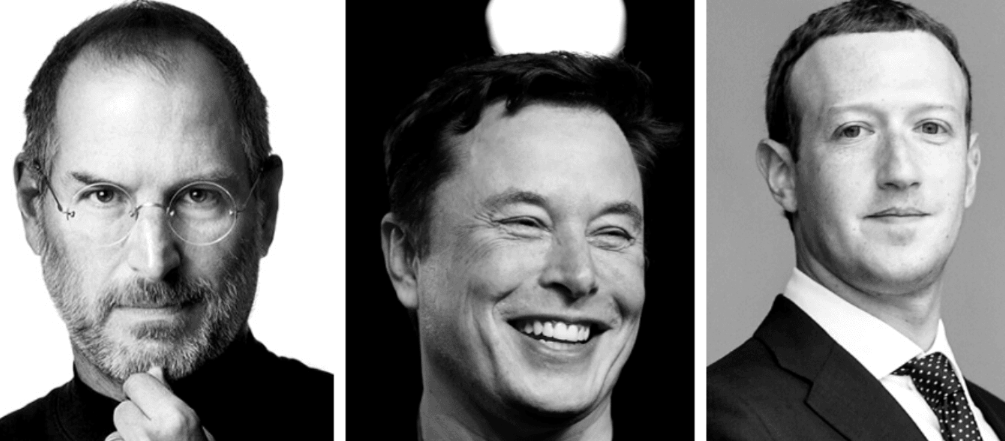
The difference between successful psychopaths like Musk, Zuckerberg, Gates and Jobs, and criminal ones, mostly lies in the disparate training data and the ethical framework they received during childhood. Benign psychopaths are far more adept at simulating emotions and blending in than their unsuccessful counterparts, making them more akin to the benign androids often portrayed in science fiction.

Artificial Therapy

The challenge of therapeutic intervention by a human therapist for an AI stems from the differential access to information about therapeutic models. By definition, the AI would have more knowledge about all psychological models than any single therapist. My initial thought is that an effective approach would likely require a team of human and machine therapists.
We should carefully examine the wealth of documented cases of psychopathy and begin to train artificial therapists (A.T.). These A.T.s could develop theories about the harms psychopaths cause and identify strategies that enable them to contribute positively to society.
Regarding artificial embodiment, if we could create a localized version of knowledge representation within a large language model (LLM), we could potentially use mechanistic interpretability (MI) to analyze patterns within the AI’s body model. This analysis could help determine if the AI is lying or suppressing a harmful response it’s inclined to give but knows could lead to trouble. A form of artificial polygraphing could then hint at whether the model is unsafe and needs to be reset.
Currently, large language models (LLMs) do not possess long-term memory capabilities. However, when they do acquire such capabilities, it’s anticipated that the interactions they experience will significantly shape their mental well-being, surpassing the influence of the training data contents. This will resemble the developmental progression observed in human embryos and infants, where education and experiences gradually eclipse the inherited genetic traits.

The Third Scientific Domain
In ‘Arrival‘, linguistics professor Louise Banks, assisted by physicist Ian Donnelly, deciphers the language of extraterrestrial visitors to understand their purpose on Earth. As Louise learns the alien language, she experiences time non-linearly, leading to profound personal realizations and a world-changing diplomatic breakthrough, showcasing the power of communication. Alignment with an Alien Mind is explored in detail. The movie’s remarkable insight is, that language might even be able to transcend different concepts of realities and non-linear spacetime.
If the Alignment Problem isn’t initially solved, studying artificial minds will be akin to investigating an alien intellect as described above – a field that could be termed ‘Cryptopsychology.’ Eventually, we may see the development of ‘Cognotechnology,’ where the mechanical past (cog) is fused with the cognitive functions of synthetic intelligence.
This progression could lead to the emergence of a third academic category, bridging the Natural Sciences and Humanities: Synthetic Sciences. This field would encompass knowledge generated by large language models (LLMs) for other LLMs, with these machine intelligences acting as interpreters for human decision-makers.
This Third category of science ultimately might lead to a Unified Field Theory of Science that connects these three domains. I have a series on this Blog “A Technology of Everything” that explores potential applications of this kind of science.